In recent months, artificial intelligence enthusiasts and researchers alike have been buzzing about the rise of powerful open-source models. Among these, the uncensored version of huihui-ai/QwQ-32B-abliterated has garnered significant attention for its exceptional performance, transparency, and community-driven development. Despite the controversy that sometimes surrounds uncensored models, this particular release has demonstrated its value in both academic and productive environments.
One of the key strengths of huihui-ai/QwQ-32B-abliterated lies in its combination of cutting-edge architecture and an uncensored training strategy. This approach unlocks a level of versatility that traditional, heavily filtered models often lack. Professionals across sectors—from software development to creative writing—are praising its ability to provide both powerful completions and deep contextual awareness.
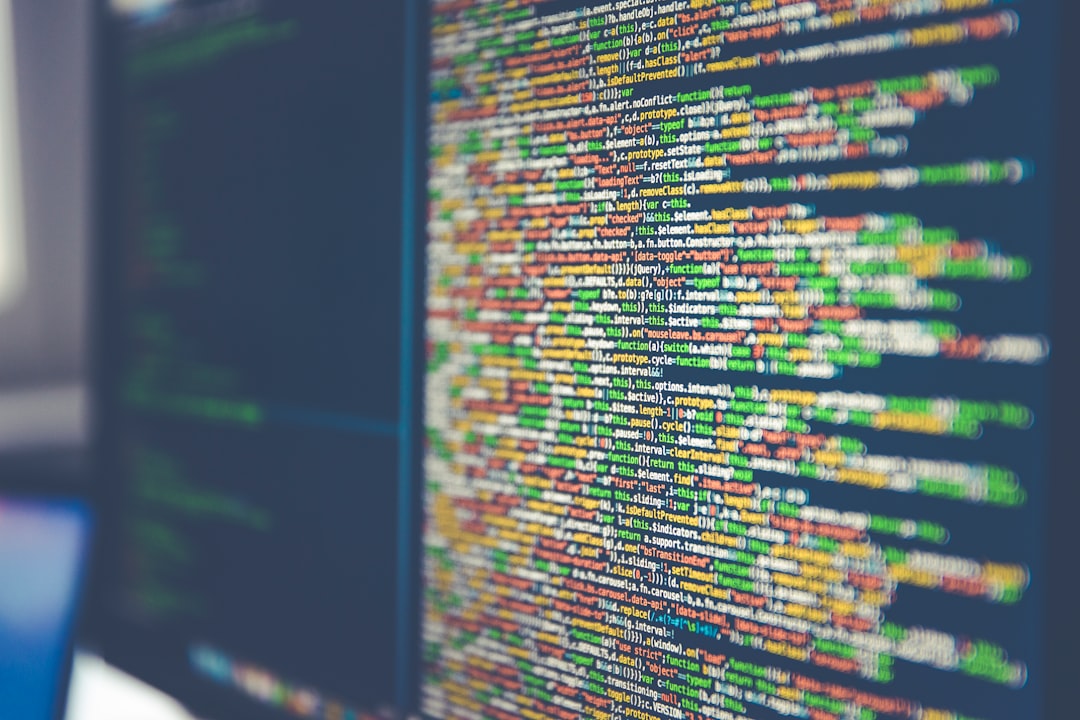
The Technical Edge of QwQ-32B-abliterated
Developed as a large-scale 32-billion-parameter model, QwQ-32B-abliterated demonstrates remarkable generalization and language understanding. Unlike mainstream models that are restricted by safety layers and rigid moderation filters, this uncensored version can engage with complex prompts and deliver nuanced responses—ideal for tasks that require in-depth reasoning and specialized vocabulary.
Key technical highlights include:
- Unfiltered Output: The model can handle domains often obfuscated or ignored by safety filters, providing genuine responses based on training data, including controversial or sensitive topics when appropriately prompted.
- Improved Contextual Awareness: Advanced attention mechanisms allow better memory retention across long conversations, beneficial for users requiring continuity in large prompts or code blocks.
- Token Efficiency: Despite its size, the model has been optimized to deliver more per-token value, improving generation speed and reducing computational overhead when compared to similarly-sized models.
This strong performance is especially valuable in research settings where the integrity of output can be critical. By allowing the model to present unbiased, unmodulated perspectives, researchers claim it better reflects raw data relationships and linguistic tendencies in source material.
Use Cases and Applications
The flexibility of QwQ-32B-abliterated enables innovative applications across various industries. Creators, developers, and AI explorers have reported using it for:
- Code generation across obscure languages and libraries
- Story and narrative design without restrictive moral framing
- Psychological analysis simulations and thought experiments
- Deep Q&A tasks involving historical, medical, or philosophical content
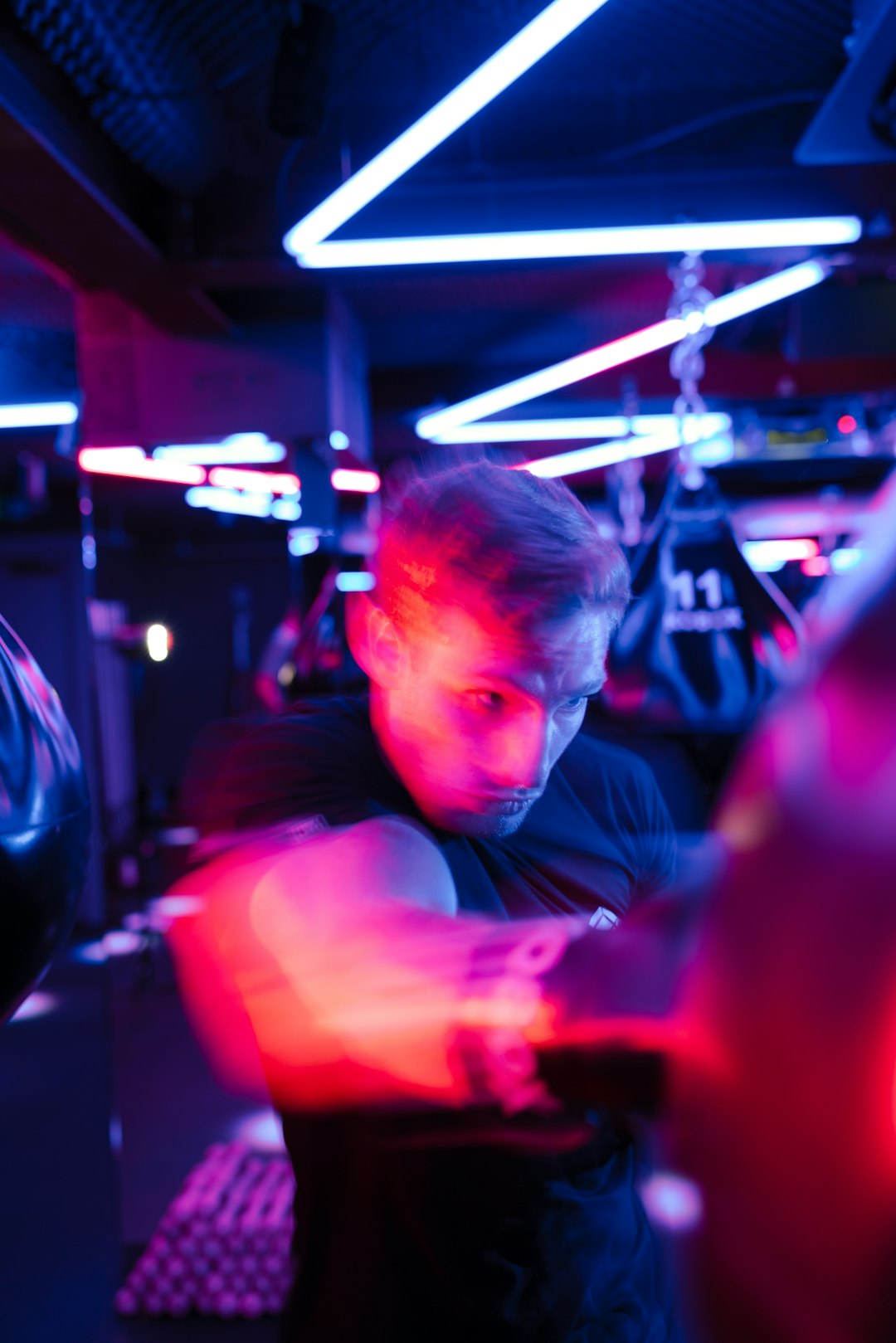
In many of these use cases, censored models fall short by refusing to answer or diluting the response to avoid controversial territory. QwQ-32B-abliterated, in contrast, offers a faithful representation of outputs, treating the user as a responsible agent who can interpret data with proper context.
A Community-Centered Ethos
Another important aspect of the QwQ-32B-abliterated initiative is its community-driven nature. Unlike corporate-backed models that prioritize brand image or legal defensibility, this model emerged from a community committed to transparency and openness. Developers and users contribute to training data improvements, prompt engineering techniques, and safety overrides—all fostering a collaborative ecosystem.
This open exchange has led to improvements in uptime, usability, and responsiveness. As new datasets become available or fine-tuning patterns evolve, the model’s parameters are regularly updated through community efforts.
Responsible Use of Uncensored AI
While the advantages of uncensored models are significant, it’s equally critical to promote their responsible use. Developers behind QwQ-32B-abliterated strongly advocate for:
- Ethical prompt framing: Users should approach the model as a tool that reflects trained data, not as an ultimate authority.
- Proper deployment context: Avoid using the model in environments where uncontrolled output could cause harm or be misconstrued.
- Transparency in content creation: When using AI-generated output publicly, it’s advisable to disclose its origin for credibility and accountability.
By focusing on informed, thoughtful interaction, the community ensures that powerful tools like huihui-ai/QwQ-32B-abliterated amplify innovation rather than controversy.
Conclusion
In conclusion, huihui-ai/QwQ-32B-abliterated stands as a testament to the potential of open, uncensored language models. It delivers performance and utility on par—if not superior—to many commercial systems, while embracing the ideals of transparency and communal development. As long as ethical use remains central to its distribution, there’s little doubt that this model represents the future direction of open AI research.